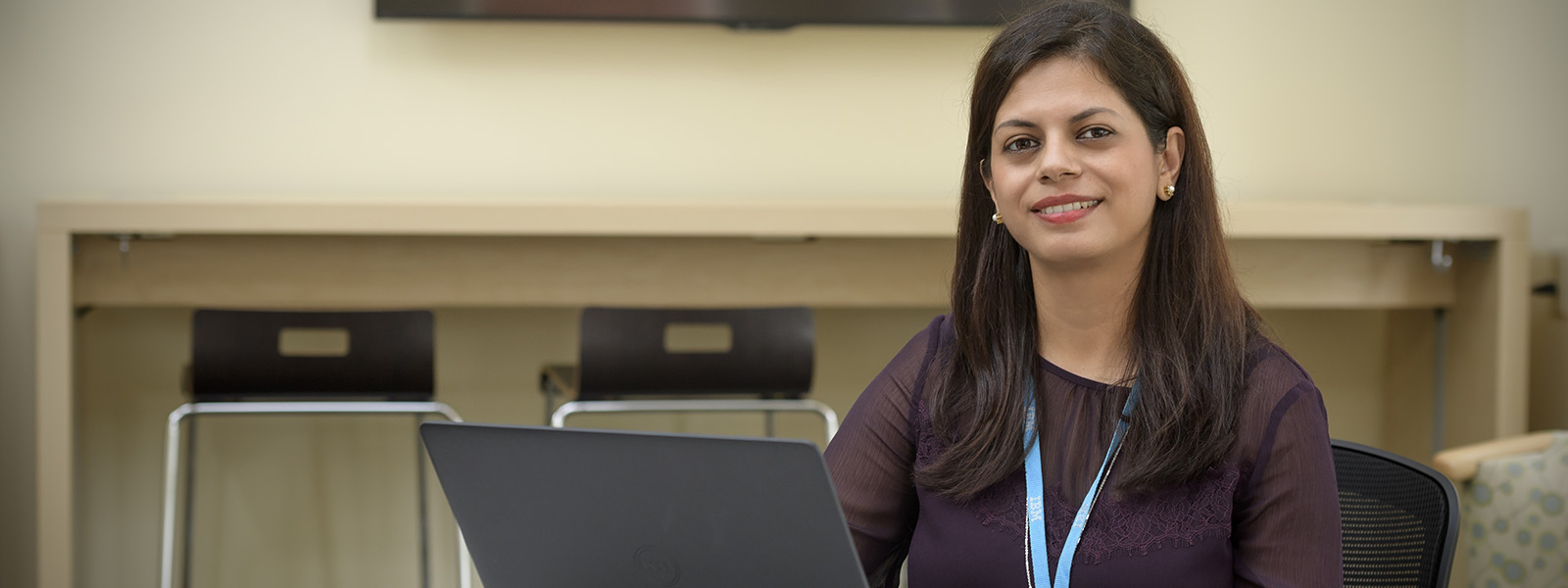
Using data-supported solutions for improving business performance
Understand why predictive analytics and data mining are so important to improving today’s business decisions
- Join the rapidly growing predictive analytics field to move from raw data to better business understanding and decision-making
- Geared toward professionals from a variety of backgrounds, including anyone who deals with large amounts of data
- 15-week in-person course — September 12-December 14 — CANCELED
- Discounts, payment plan, scholarships available
- LEARN MORE — view info session presentation
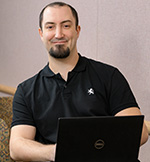
In today’s business world, data is easier than ever to collect and store. While the management of this big data is increasingly important to the decision-makers in the organization, big data is ever more difficult to analyze.
Analytics professionals or data-mining experts are invaluable to an organization’s success. They have the unique combination of computational, analytical and communication skills necessary to discover data-supported solutions to important business questions, from an ever-increasing wealth of data.
This program introduces students to the tools needed to analyze diverse kinds of data in order to make more informed business decisions. Students learn to gather and organize data for more effective analysis and how to communicate their analyses in a clear and concise manner.
Along with analyzing data from apps and fitness trackers to help stop the spread of COVID-19, data science professionals have been using big data to predict patient treatment outcomes, monitor patients as they enter hospitals, identify promising drug candidates, estimate real-time spread and forecast future spread of the coronavirus. – LEARN MORE
Program details
Predictive Analytics and Data Mining Certificate – Noncredit Certificate
LOCATION: Arsht Hall – Wilmington, Delaware
SCHEDULE: September 12-December 14, 2022 — Mondays, 6-9:15 p.m. (Class meets twice in the final week on Monday and Wednesday) – CANCELED
PRICE: $2,895, Payment plan, scholarships and potential discounts available, including: Elevate Delaware funding, Early registration, Military, UD student or alum, 2 or more (group). Optional textbook additional: JMP® Start Statistics: A Guide to Statistics and Data Analysis Using JMP®, Sixth Edition, John Sall, Ann Lehman, Mia Stephens, Sheila Loring. ISBN# 978-1-62960-875-4.
CEUs: 4.5 (45 contact hours)
- Who should participate in this program
- Instructors
- Course outline
- Learner outcomes
- NEW! Career resources to help you succeed
- Technology requirements
- Prerequisites
- What our students say
- For more information
Who should participate in this program?
- Business, marketing and operations managers
- Data analysts or professionals in any field who deal with large amounts of data
- Healthcare industry professionals
- Financial industry professionals
- Anyone who wishes to learn how to use historical data to predict future outcomes
Consider these applicable use cases:
- Predicting employee participation in healthcare insurance: Companies offer health insurance to attract and retain talent, and they often subsidize the premiums to make coverage more affordable. But what is the return on that investment with respect to retention (length of service)? And what impact, if any, would an increase in an employer’s premium subsidy have on length of service? Logistic regression and decision tree modeling were done to identify factors that best predict whether an employee will elect certain health insurance options. The results of these models help to budget for acquisitions and business expansion.
- Predicting diabetes: A major study of diabetes was carried out at several hospitals. The purpose of this project was to determine whether a short questionnaire containing yes/no answers to a small number of questions (plus age in years) generated by a patient in consulting with a physician can predict if that patient has diabetes. After collecting data and using different models, it was found that using a partial least squares analysis can predict the diagnosis with about 85% accuracy. Using a neural network analysis with three hidden units gives 100% accuracy (no prediction errors) for all cases.
- Identifying source of product performance problems: Several key customers of a manufacturing company have noticed a deviation in product performance from what they normally obtain from that company. This manufacturing company pulled together a database of recent history of raw-material, in-process and final-product variables on the data-mining tools that were applied to identify the source of these product deviations.
- Predicting competitive bond buying bids: A financial organization insures municipal bond deals. It wants to underbid its competitors on each deal, as a modest increase in performance is worth over $3 million per year. The goal is to be the lowest bidder, but not by much, so that little is left on the table. Data on the 100-plus most recent deals were collected, with various financial performance indicators as inputs and the winning competitive bid as output. Regression analysis was used to develop a predictive model to use for future deals.
- Identifying crowdsourcing success factors: What elements provide the greatest influence on a successful Kickstarter project? Data-mining tools were applied to one year of data sampled from the “Board and Card Games” category of the Kickstarter database. Four recurring variables were found to be significant when cross-referencing the methods (regression analysis, neural networks and decision trees) that were used to analyze this data.
Instructors
Steven P. Bailey was with DuPont’s corporate Applied Statistics Group for over 36 years until his retirement as a principal consultant in 2016. During his last 16 years with DuPont, Bailey led DuPont’s corporate Six Sigma Master Black Belt Network. A past president and chairman of the board of the American Society for Quality (ASQ), he is certified as a Six Sigma Black Belt and Master Black Belt by both DuPont and ASQ.
Bailey, who served as an adjunct faculty member in UD’s Department of Applied Economics and Statistics, has been an instructor for the Predictive Analytics and Data Mining Certificate program since 2012. He also provides statistics and Six Sigma training and consulting services for a variety of businesses. He earned his B.S., M.S. and doctorate in statistics at the University of Wisconsin in 1974, 1975 and 1979, respectively.
Aaron J. Owens retired in 2015 as a senior research fellow with the Decision Analytics Group at the DuPont Company. The group uses analysis of diverse types of data as well as quantifying uncertainties to aid in making important business decisions.
Owens holds a B.S. with highest honors in physics from Williams College (1969) and an M.S. (1971) and a doctorate (1973) in theoretical physics from Caltech. Following several years of teaching physics, astrophysics and mathematics and conducting astrophysics research at Lake Forest College, Kenyon College and the University of Delaware, he joined DuPont in 1980. Owens specialized in mathematical modeling of chemical systems, color modeling and supercomputing, and was the technology leader of applied statistics. He founded DuPont’s data-mining efforts, developed the company’s proprietary neural network technology and adopted chemometric methods for process modeling. Currently, he does freelance consulting on data mining, chemometrics and applied statistics for the chemical and pharmaceutical industries.
Course outline
Topics include:
- Importing data into an analytics software package
- Performing exploratory graphical and data analysis
- Building analytics models, also called data mining, using tools such as multiple regression and machine learning tools like artificial neural networks and decision trees
- Finding the best model to explain correlation among variables
- Learning how to control and assess data variability to better meet customer requirements
Grading policy — To earn the Predictive Analytics and Data Mining Certificate, students must complete each of the four modules with a passing grade of 60 or higher and earn an overall final grade of 70 or higher:
- Analytics Basics
- Machine Learning and Data-Mining Tools, including for Big Data
- Process Control and Capability
- Individual Project
Learner outcomes
Analytics Basics
In this module, students will be introduced to the basics of analytics by learning key terms, concepts and knowledge areas and the use of SAS JMP Pro analytics software. At the end of this module, students will be able to:
- Navigate JMP software
- Input data from various spreadsheets and databases
- Perform graphical exploratory analysis
- Use analysis of variance to compare multiple groups of data
- Use regression analysis to predict relationship between continuous inputs and outputs
- Use contingency table analysis to quantify relationships between categorical variables
- Use logistic regression to predict categorical outcomes from one or more inputs
Machine Learning and Data-Mining Tools, including for Big Data
In this module, students will learn how to use machine learning and big data tools to understand correlation among many different variables. At the end of this module, students will be able to analyze large datasets and build analytical models to predict future performance using the following multivariate tools:
- Multiple regression
- Discriminant analysis
- Cluster analysis and market segmentation
- Principal component analysis and partial least squares
- Artificial neural networks
- Decision trees
- Support vector machines
- Multivariate time series analysis
Process Control and Capability
In this module, students will learn how to evaluate if a process is stable and its ability to meet customer requirements. Students will also learn how to evaluate a measurement system’s performance and variability. At the end of this module, students will be able to use the following tools:
- Process control charts
- Process capability analysis
- Modeling process variation
Individual Project
As a final requirement of the certificate, students will apply the concepts and techniques learned throughout the course to a case study project. The project will incorporate the use of a current data problem the student would like to solve, which will be approved by the instructor. Guidance in completing project milestones is done throughout the program.
Career resources to help you succeed
NEW! — Participants enrolled in this certificate program will have access to a new suite of career resources and services to help them navigate a career transition, maximize their job search efforts and more. Click here to learn more about UD PCS Career Services.
Technology requirements
- A laptop or desktop computer (PC or Mac) with Microsoft Excel is required to participate in this class.
- A copy of JMP® Pro statistical analysis software is included with this program, and the software is compatible with both Mac and PC.
Prerequisites
While there are no formal requirements for admission to this program, please note the following recommendations:
- Statistics background—The course uses statistics throughout the curriculum. A prior college level statistics course and/or working knowledge of statistics is required.
- Some prior college coursework is recommended, as the modules are taught at the baccalaureate level and are fairly rigorous.
- Prior experience with computer-assisted data management is helpful.
- Study and class preparation—Most students have said that for every hour in class they spent two hours outside of class studying and preparing for class, as well as additional time for work on the final project.
- JMP® Pro statistical analysis software will be taught and used in the classroom.
What our students say
- “I didn’t have the confidence to go for my master’s in data science until I went through the classes, did the work and realized that I could indeed do this.” – Jamie Spencer
- “I am definitely implementing what I learned at UD into my work” – Sambhavi Parajuli
- “The ability to think conceptually about big data has been enormously helpful in transitioning to a new job that I was able to get during the course of the program.” – Patrick Caruso
- “This course has gone a long way in simplifying my workload and showing quick results to my internal customers.” – Amogh Prabhu
- “There is an explosion of data that can be found in every industry and government agency around the globe. The trick is to be able to take this data and turn it into information to drive better decisions.” – Joe Messick